Our Research
Understanding Remote Sensing Images
The automatic extraction of map information, such as land cover labels and elevation models from remote sensing imagery, is our core project. Machine learning is a powerful tool to automate mapping, but the quality and quantity of training data are the key. We build world-leading benchmark datasets for land cover mapping addressing geographic equity [Xia+, WACV'23] [Song+, WACV'24]. We also work on mapping from incomplete training data [Li+, JSTARS'22]. How to recognize changes from images of different modalities and automatically update maps is also a key challenge [Chen+, TGRS'22].
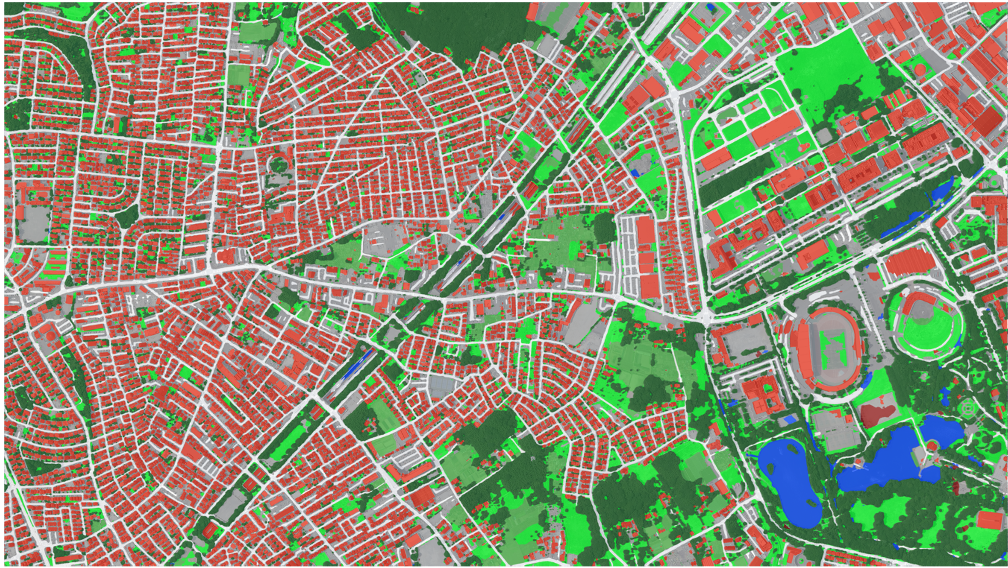
Solving Inverse Image Problems
We work on solving image inverse problems, such as compressive spectral imaging, super-resolution, denoising, and inpainting to obtain high-spatio-spectral-temporal-resolution images that exceed hardware limitations. We develop algorithms based on deep learning and matrix and tensor decomposition that contribute to the generation of higher-order products from airborne and satellite data. Our recent work include cross-modal super-resolutioin [Dong+, ECCV'22] and neural architecture search for compressive spectral imaging [He+, ECCV'22].
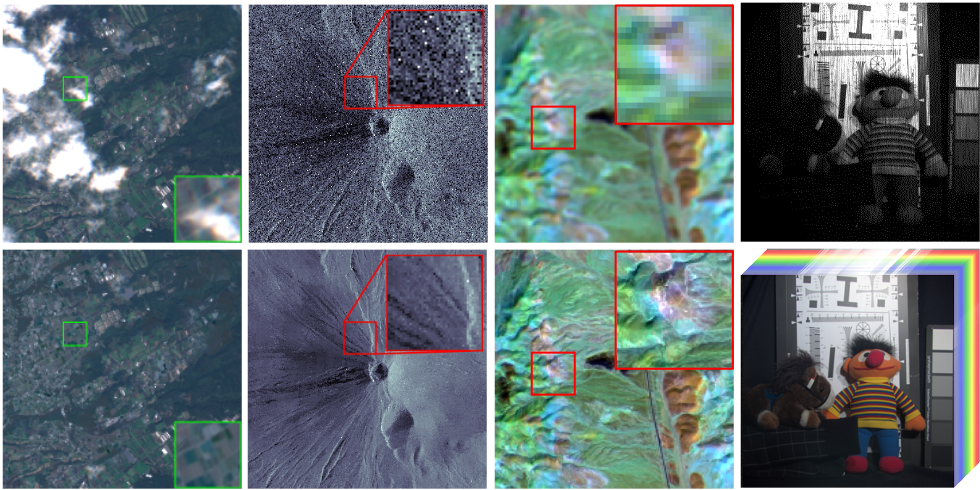
Toward a Sustainable Future
Our research is grounded in solving global issues toward, such as climate change, large-scale natural disasters, and food problems. Our recnet work includes 3D change (e.g., inudation depth and terrain deformation) estimation during disasters [Yokoya+, TGRS'22], biomass and carbon stocks estimation in mangroves [Le+, IJRS'21], and crop type classification [Xia+, IJAEOG'23], in collaboration with related institutions and researchers in Japan and overseas. In real-world problems, the lack of training data is a more serious issue. A unique feature of our approach is the successful use of heterogeneous data from different disciplines as training data.
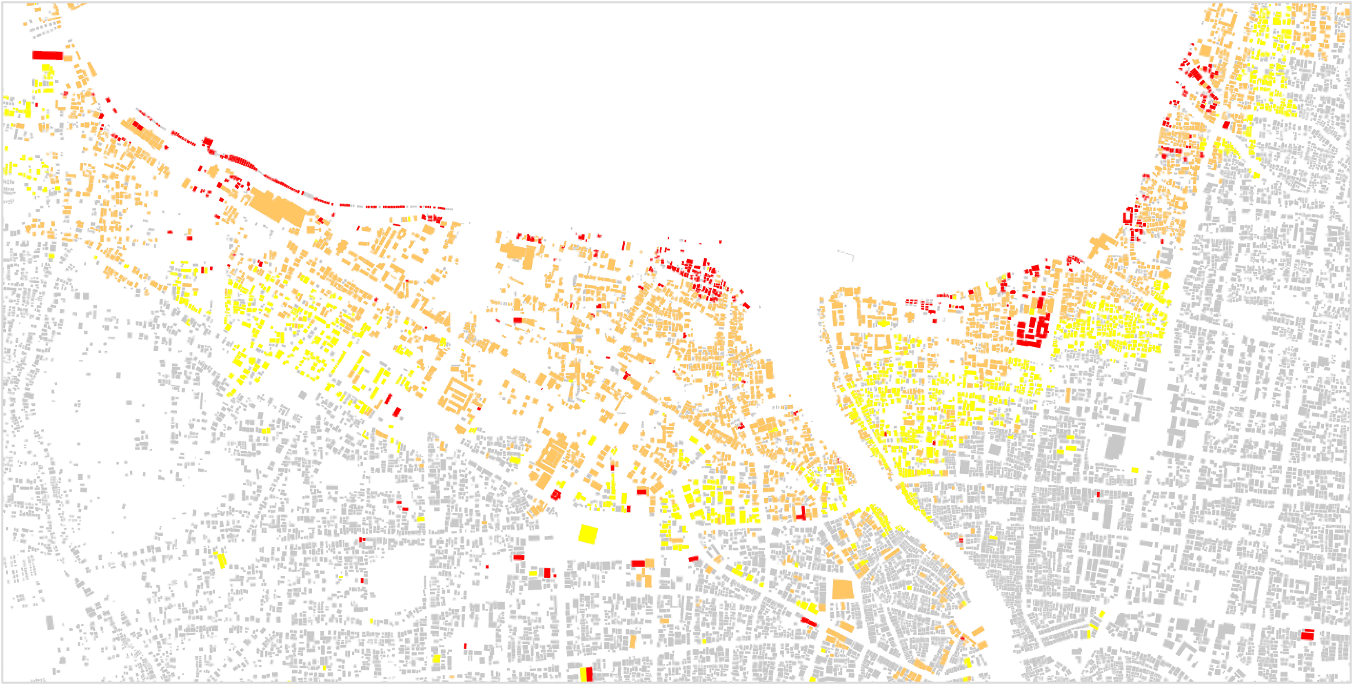